A data library to strengthen external data value
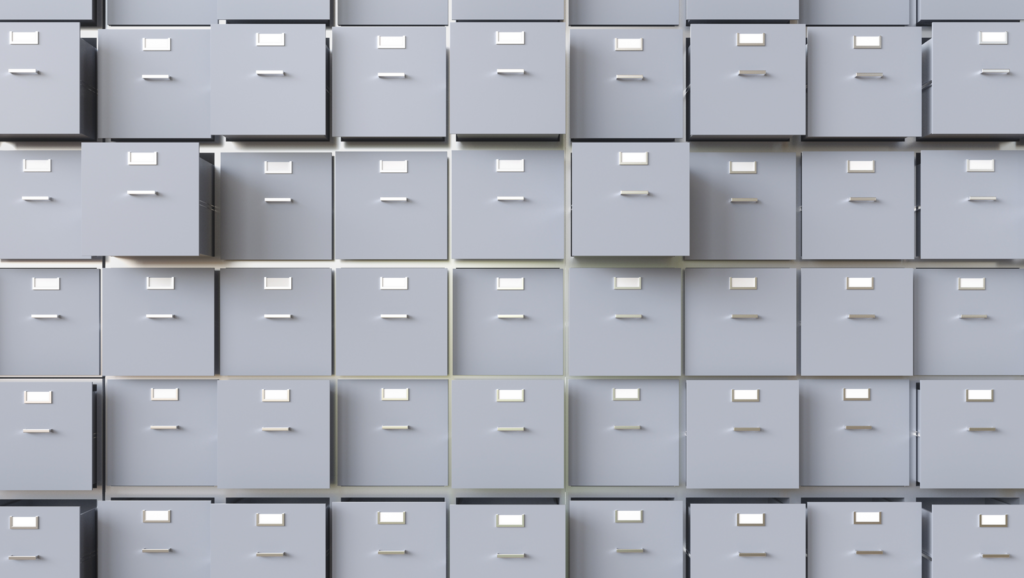
Private and public open data, social network data, private data platforms… The web is an infinite source of external data. It comes in a variety of formats: data tables, geolocated data, APIs, images and text. How can organisations take advantage of all the value to be gained from big data processing?
Before you hear about namR’s solutions, it’s important to understand a bit about what they do. namR is a data producer that uses only external data in its data science processes. This unique founding principle has one important advantage — no reliance on data from partners who enforce data exclusivity/protections preventing data use. namR has extensive expertise with data in every sector of the ecological transition: renewable energy development, energy efficiency operations, smart grids, short circuits . . . Its data science teams exploit not only geolocalised data, but also images and textual corpora to build an incredibly fine mesh of actionable information for a wide variety of actors.
Given that external data is namR’s only source of data, they focus on exploitation to the fullest extent. This is why the start-up has tasked itself with building the widest possible structured knowledge base.
The first requirement of this database was that it be comprehensive, drawing from every structured data source in France. Exhaustive research into open and closed data sources was crucial, and monitoring efforts are ongoing. namR developed scrapers that browse the pages of these sources on a daily basis. The scrapers download available datasets and retrieve the metadata in a structured way.
The second requirement was to harmonize the information available on each of the databases so that queries would be evenly distributed. This meant developing data mining tools that complete the work of the scrapers by browsing the downloaded files. The scrapers extract a vast array of information from each of the files: number of records, number of variables, column headers and types, and very soon they will reveal single or multiple themes thanks to an algorithm of Natural Language Processing.
Finally, the third requirement was to set up a fluid pipeline integrating external data into machine learning processes. The robustness of the pipeline is based on its ability to adapt to source data updates. Upon receiving an alert form the scrapers, the data scientist can update the databases upstream of the flow. In the short term, the Data Library will be able to score evolutions resulting from dataset updates. If the schema remains consistent and the number of records is not increased tenfold, the dataset will be automatically updated.
The open data movement and the multiplication of data marketplaces both present opportunities that can only be seized with new tools. The namR Data Library is equal to the challenge. Although the library is still in development, it already fulfils several internal functions. Its first public trial run will be in February as part of the open data observatory co-developed by namR, OpenData France, Etalab and the Cour des Comptes.